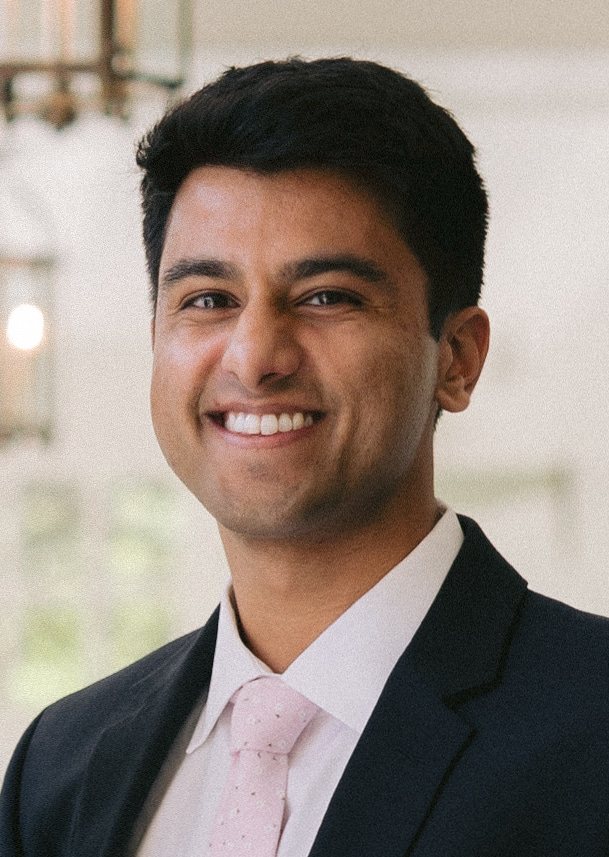
Hi! I'm currently a Ph.D student at UC Berkeley, where I'm advised by Sanjit Seshia. My Ph.D. has been gratefully supported by the NDSEG Fellowship.
My research interests lie broadly at the intersection of machine learning and formal methods, with particular focus on applications in robotics, autonomy, and program synthesis. By applying formal reasoning to learning algorithms, I hope to make real-world ML systems more trustworthy, compositional, and interpretable.
I received my Bachelor's and Master's degrees from Rice University, where I worked on projects related to interpretable machine learning via program synthesis and formal methods. I was advised by Swarat Chaudhuri, who I worked with on the "Understanding the World Through Code" NSF Expeditions Project. As an undergrad, I also collaborated with Ankit Patel and Richard Baraniuk. I was fortunate to be supported by the Rice CS Graduate Fellowship.
I've spent time at Microsoft Research AI, where I worked with Alex Polozov and the GRAIL group on interactive ML-driven program synthesis. I also collaborated with Jon DeCastro and the Human-Aware AI team at TRI to improve learned behavior in safety-critical settings.
In my free time, I enjoy doing a lot of things, including but not limited to: playing tennis, reading novels, cooking, and repeatedly fixing and breaking my jumpshot. I'm originally from Cleveland, Ohio, and I love watching my beloved Cavaliers break my heart year after year.
You can find me on X or on Google Scholar. The best way to reach me is by sending me an email at ameesh@berkeley.edu.
Recent News (see all)
Publications
Conference Papers
in Autonomy-to-Human Perception Hand-offs
Automata and Recurrent Neural Networks
Preprints and Technical Reports
Aware Imitation Learning